
Radiology study seeks fuller picture of 'incidentalomas'
Imaging scans that incidentally detect masses – cancerous and benign – lead to follow-up appointments, costs and worry.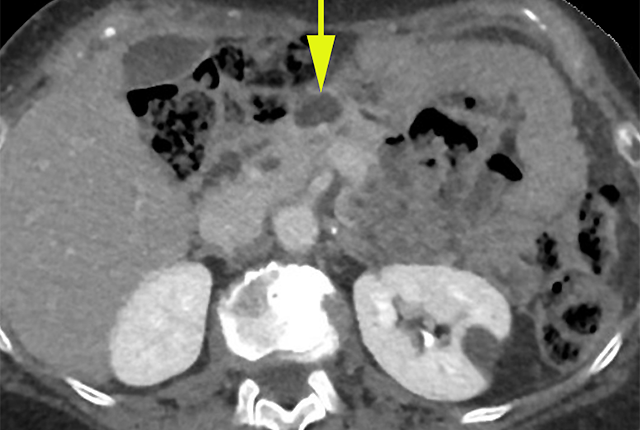
Say you get a CT scan to confirm whether your chest pain is from a fractured rib. You learn a few days later that no bone break was detected – yay! – but joy gives way to anxiety as you read the radiologist’s note, from the same scan, of a spot in one of your lungs.
With advances in medical imaging and our aging population, such findings are increasingly common. Doctors call them “incidentalomas.” Although in most cases the attendant risk is low, sometimes they represent very early cancers. Patients can, of course, be consumed with dread at the suggestion of cancer and the financial hit of recommended follow-up scans and biopsies.
“These apparent cancers have a wide spectrum of appearances. When we identify them, we usually don’t know whether they represent a cancer or whether there is short- or long-term risk,” said Dr. Martin Gunn, a professor of radiology at the University of Washington School of Medicine. “We usually recommend follow-up with advanced imaging like ultrasound, CT, MRI, or nuclear medicine to find out and to ensure it does not grow.”
Gunn will co-lead a just-funded study of incidental findings to shed light on which patients and imaging findings put patients at the highest and lowest risk of adverse outcomes. He’ll work with Meliha Yetigsen, UW associate professor of biomedical informatics.
The project will analyze 4 million radiology reports to gauge incidentalomas’ impact on morbidity, mortality, and the cost-effectiveness of recommended imaging surveillance and intervention.
The researchers will examine the six most frequently detected incidental lesions: in the lung, liver, kidney, pancreas, adrenal gland, and thyroid gland. They will develop a database of individual records detailing the follow-up costs involved, and ultimate result – a benign or cancerous finding, a “lost” case in which the patient does not return for subsequent care, and whether the incidentaloma resulted in poor health outcomes. Each record would also identify comorbidities and demographic info that might lend insight to risk factors and social determinants of the patient’s long-term health, Gunn said.
“If we look at lung nodules, in the low-risk person with an incidental nodule of less than 6 millimeters, the risk of that being cancer is less than 1%. In that setting we’d only recommend follow-up if the patient has a strong smoking history. At the other end of the spectrum, if the lung nodule is more than 1 centimeter, the cancer risk is substantially higher,” Gunn said. “So we’re hoping to better identify which cases are most appropriate to follow up, and what’s the best way to do that.”
Yetisgen is the project’s lead data miner. She will design software that recognizes and gathers meaningful information from the free text of radiologists’ clinical reports and notes.
“We sampled 500 CT notes to create a schema that defines what information we’re trying to extract, say, tumor findings,” Yetisgen said. “We’ll manually label descriptors, like “lesion” and “malignant” and references to sizes like millimeters and centimeters in these subsets of information. Then we will build a software language model that will automatically learn the lexicon associated with those labels.”
The model’s accuracy is crucial, so it will need to be trained and tested multiple times until it can be validated as representative of the full 4 million records.
The task may sound daunting, but Yetisgen pointed out one upside: “Radiology reports follow a standard and usually are quite nicely structured, so they are much simpler than patient admission notes or discharge notes, which can differ widely even at the same institution,” she said.
The four-year research project is being funded with an award (R01CA248422) of more than $2 million from the National Cancer Institutes, part of the National Institutes of Health.
For details about UW Medicine, please visit http://uwmedicine.org/about.