
Accurate protein structure prediction now accessible to all
New artificial intelligence software can compute protein structures in 10 minutes.Media Contact: Leila Gray, 206.475.9809,leilag@uw.edu
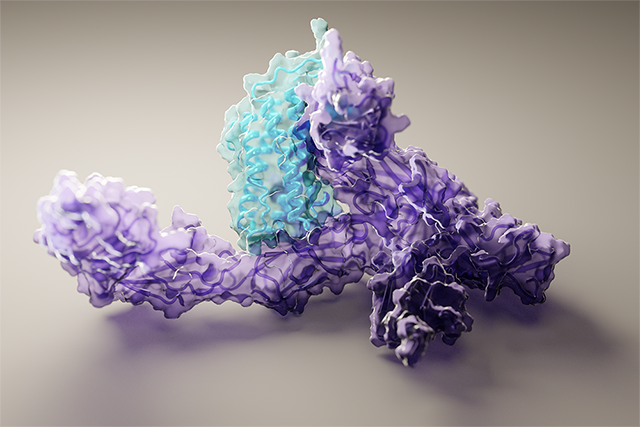
Scientists have waited months for access to high-accuracy protein structure prediction since DeepMind presented remarkable progress in this area at the 2020 Critical Assessment of Structure Prediction, or CASP14, conference. The wait is now over.
Researchers at the Institute for Protein Design at the University of Washington School of Medicine in Seattle have largely recreated the performance achieved by DeepMind on this important task. These results will be published online today, July 15, by the journal Science. Here is the paper.
Unlike DeepMind, the UW Medicine team has already made their method, dubbed RoseTTAFold, freely available. Scientists from around the world are now using it to build protein models to accelerate their own research. Soon after its recent upload, the program was downloaded from GitHub by over 140 independent research teams.
Proteins consist of strings of amino acids that fold up into intricate microscopic shapes.These unique shapes in turn give rise to nearly every chemical process inside living organisms. By better understanding protein shapes, scientists can speed up the development of new treatments for cancer, COVID-19, and thousands of other medical disorders.
“It has been a busy year at the Institute for Protein Design, designing COVID-19 therapeutics and vaccines and launching these into clinical trials, along with developing RoseTTAFold for high accuracy protein structure prediction. I am delighted that the scientific community is already using the RoseTTAFold server to solve outstanding biological problems,” said senior author David Baker, Howard Hughes Medical Institute Investigator, professor of biochemistry, and director of the Institute for Protein Design at UW Medicine.
In the new study, a team of computational biologists led by Baker developed a software tool called RoseTTAFold that uses deep learning to quickly and accurately predict protein structures based on limited information. Without the aid of such software, it can take years of laboratory work to determine the structure of just one protein.
RoseTTAFold, on the other hand, can reliably compute a protein structure in as little as 10 minutes on a single gaming computer.The team used RoseTTAFold to compute hundreds of new protein structures, including many poorly understood proteins from the human genome. They also generated structures directly relevant to human health, including for proteins associated with problematic lipid metabolism, inflammation disorders, and cancer cell growth. And they show that RoseTTAFold can be used to build models of complex biological assemblies in a fraction of the time previously required.
RoseTTAFold is a “three-track” neural network, meaning it simultaneously considers patterns in protein sequences, how a protein’s amino acids interact with one another, and a protein’s possible three-dimensional structure. In this architecture, one-, two-, and three-dimensional information flows back and forth, allowing the network to collectively reason about the relationship between a protein’s chemical parts and its
folded structure.
“We hope this new tool will continue to benefit the entire research community,” said
Minkyung Baek, a postdoctoral scholar who led the project in the Baker laboratory at UW Medicine.
This work was supported in part by Microsoft, Open Philanthropy Project, Schmidt
Futures, Washington Research Foundation, National Science Foundation, Wellcome
Trust, and the National Institute of Health. A full list of supporters is available in the Science paper.
-- written by Ian Haydon, UW Medicine Institute for Protein Design
For details about UW Medicine, please visit http://uwmedicine.org/about.