
Deep learning tool identifies bacteria in micrographs
Omnipose is trained to recognize bacteria of all shapes and sizes in mixed bacterial cultures.Media Contact: Leila Gray, leilag@uw.edu
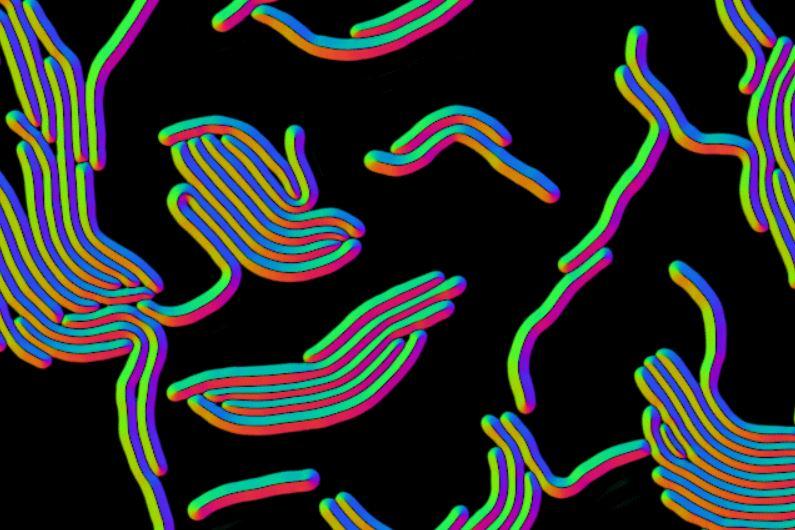
Omnipose, a deep learning software, is helping to solve the challenge of identifying varied and miniscule bacteria in microscopy images. It has gone beyond this initial goal to identify several other types of tiny objects in micrographs.
The UW Medicine microbiology lab of Joseph Mougous and the University of Washington physics and bioengineering lab of Paul A. Wiggins tested the tool. It was developed by University of Washington physics graduate student Kevin J. Cutler and his team.
Mougous said that Cutler, as a physics student, “demonstrated an unusual interest in immersing himself in a biology environment so that he could learn first-hand about problems in need of solution in this field. He came over to my lab and quickly found one that he solved in spectacular fashion.”
Their results are reported in the Oct. 17 edition of Nature Methods.
The scientists found that Omnipose, trained on a large database of bacterial images, performed well in characterizing and quantifying the myriad of bacteria in mixed microbial cultures. It also eliminated some of the errors that can occur in its predecessor, Cellpose.
Moreover, the software wasn’t easily fooled by extreme changes in a cell’s shape due to antibiotic treatment or antagonism by chemicals produced during interbacterial aggression. In fact, the program showed that it could even detect cell intoxication, or poisoning, in a trial using E. coli.
In addition, Omnipose did well in overcoming recognition problems due to differences in the optical characteristics across diverse bacteria.
Most bacteria are spheres or rods, but some have other basic forms, such as twisting spirals. Besides these, Omnipose could identify more elaborate bacteria with elongated shapes or with branches, filaments and appendages, all physical traits that can make it difficult for deep learning tools to determine which bacteria are present in an image.
The program does still face some limitations in handling object overlap in a 2D rendition of a 3D sample of a crowded microbial community. Object overlap is what produces, for example, the effect of a clock on a wall giving the illusion of popping out of a person’s head in a photograph.
In analyzing cells in a Omiroot primordial data set from the fast-growing weed A. thaliana, Omnipose nonetheless did show some advantages over previous approaches in this 3D sample.
Other reviews by the Mougous lab team of Omnipose’s capabilities showed bacteria below a certain threshold in size can be hard for the tool to suss out.
Despite these drawbacks, the researchers believe that Omnipose could be a solution, they noted, to “help answer diverse questions in bacterial cell biology.”
To see if it could also become a multifunctional tool in other biological or even non-life sciences fields dependent on microscopy, the scientists tried out the program on micrographs of the ultra-tiny roundworm C. elegans, an important organism in genetic, neuroscience, developmental and microbial behavior research. Like some bacteria, this creature has an elongated shape. Like many other worms, it also can contort itself. Omnipose could pick out C. elegans regardless of its various stretches, contractions, and other movements. This ability could be useful, for instance, in neural studies of C. elegans locomotion during time-lapse tracking.
In designing tools like Omnipose, researchers are looking at a scale of single-pixel precision to define the boundaries of a cell. That’s because most bacterial cell body images are composed of only a small number of pixels. The researchers explained that defining boundaries within an image is called segmentation. They developed Ominpose through a deep neural-network, high precision segmentation algorithm. Their experiments showed Omnipose has an unprecedented segmentation accuracy.
The scientists designed Omnipose for use by typical research laboratories and made its source code, training data and models publicly available, along with documentation on how to use the program.
“We anticipate that the high performance of Omnipose across varied cellular morphologies and modalities,” the researchers wrote in their report, "may unlock information from microscopy images that was previously inaccessible.”
“Reflecting the importance of the problem, this is a crowded field,” Mougous said. “Yet Kevin’s solution stands out from the pack. We believe it will be a game-changer for biological image analysis.”
In addition to Cutler, Wiggins and Mougous, other researchers on the Omnipose testing project were Carsen Stringer, Teresa W. Lo, Luca Rappez, Nicholas Stroustrup. S. Brooke Peterson, and Paul Wiggins. Mougous is a Howard Hughes Medical Institute investigator.
This work was supported by the National Institutes of Health (AI080609, GM128191, R01-GM128191 and T32-GM008268), European Research Council under the European Union’s Horizon 2020 Research and Innovation Programme (852201), Spanish Ministry of Economy, Industry and Competitiveness to the EMBL partnership, Centro de Excelencia Severo Ochoa (CEX2020-001049-S, MCIN/ AEI /10.13039/501100011033), CERCA Programme/Generalitat de Catalunya and the Spanish Ministry of Economy, Industry and Q22 Competitiveness Excelencia (PID2020-115189GB-I00), and the Howard Hughes Medical Institute.
For details about UW Medicine, please visit http://uwmedicine.org/about.
Tags:microbiology