
Mammography experts caution against assuming AI’s benefit
Radiologists feel pressure to use and promote technology still unproven in population-level studies, editorial says.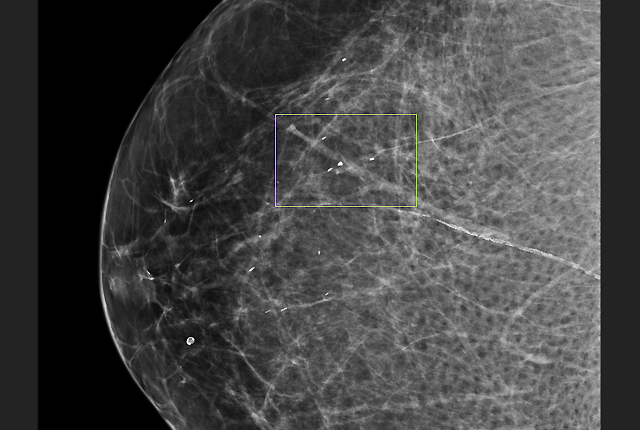
Almost weekly, new study findings proclaim the value of artificial intelligence in medicine, especially in radiology. “AI outdoes radiologists when it comes to identifying hip fractures, study shows,” a Washington Post headline said Feb. 20.
So it was intriguing when two breast cancer screening experts threw a little cold water on the AI fervor in a Feb. 25 editorial in JAMA Health Forum.
Drs. Christoph Lee, at UW Medicine, and Joann Elmore, at UCLA, co-wrote a commentary that cited troubling parallels between AI’s rapid uptake and that of computer-aided detection (CAD), a technology that radiologists hailed two decades ago for its usefulness in detecting breast cancer—that is, until a major study showed that CAD did not improve mammography accuracy.
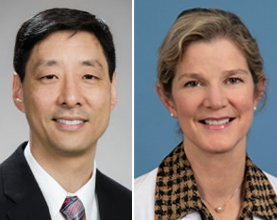
“CAD did not increase cancer detection and it increased false positives and benign biopsies at the population level,” said Lee, professor of radiology at the University of Washington School of Medicine. “This is the lesson that Joann and I are pointing to, saying that we have to do it differently this time. We cannot fall victim to the same automation bias and widespread adoption before we truly have more evidence that it improves outcomes for all women.”
CAD won FDA approval and Medicare reimbursement after early reader studies showed improved accuracy with the technology. But in 2016, a decade after those early wins had been invalidated, more than 92% of U.S. imaging facilities were still using CAD for mammography interpretation, the authors noted.
AI has followed that same early path, scoring wins in reader studies. In these studies, a radiologist goes head to head with AI, interpreting a specially prepared set of a couple hundred breast scans that have been goosed with a much higher rate of cancer findings than one would see at a population level.
“If you create a case set of 240, you probably include 100 cancer positives,” Lee explained. “In the real world, cancer-positive cases only account for about five in every 1,000 screenings we do. The radiologists involved know that the case sets include many more cancer findings than would be the case among 240 randomly collected mammogram screenings. Moreover, they know that they’re up against an artificial intelligence system. So the radiologists are more sensitive to detecting cancer.”
Reader studies are designed this way to gather the most possible evidence of AI’s cancer-detection prowess – because improved detection is what the Food & Drug Administration needs to approve an algorithm, Lee said. Unfortunately, this design also overlooks AI’s red flags, which in the real world might contribute to a false-positive reading and a patient being called back for additional tests that cause emotional and financial distress.
“Even if it detects a few cancers in complex cases, but leads to hundreds of benign biopsies, the risk-benefit tradeoff would not support these new technologies,” Lee said.
Radiology departments everywhere feel pressure to get aboard the AI train, he added.
“If one radiology group is marketing new technologies as a come-on to patients, they have a leg up. Women who are concerned about detecting disease early may prefer medical centers that have this AI radiology software available, even without any population-level evidence of risk versus benefit.”
AI must have not only its effectiveness, but its benefit, measured in large, population-based screening studies in which patients’ outcomes data are referenced over a span of years and linked to breast-cancer registries, Lee and Elmore wrote. If more benefits than harms are found, the next step would be ensuring those results are consistent across diverse populations.
It’s a knotty issue, for sure, partly because of AI’s promise to get smarter with each scan it reads. A five-year test of an algorithm’s benefit also means that, when results are being finalized for submission to a journal, the test algorithm may be outdated.
For now, industry adoption is happening apace. “The FDA approval process is a very low bar for AI software technology. They’re not set up to ensure population level effectiveness,” said Lee.
For details about UW Medicine, please visit http://uwmedicine.org/about.