
Study: AI improves radiologists’ readings of mammograms
In a study of breast-cancer screening accuracy, adding artificial intelligence to experts’ opinion had favorable results.Media Contact:
- (UW School of Medicine) Brian Donohue - 206.543.7856, bdonohue@uw.edu
- (IBM Research) Kristi Bond - 802.345.8313, kristi.bond@us.ibm.com
- (Sage Bionetworks) Hsiao-Ching Chou - 206.696.3663, chou@sagebionetworks.org
- (Kaiser Permanente) Rebecca Hughes - 206.287.2055, rebecca.f.hughes@kp.org
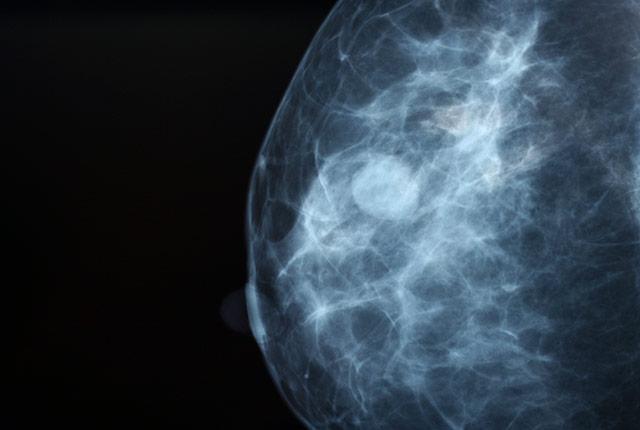
Machine-learning algorithms could help improve the accuracy of breast cancer screenings when used in combination with assessments from radiologists, according to a study published today in JAMA Network Open.
The study was based on results from the Digital Mammography (DM) DREAM Challenge, a crowd-sourced competition to engage an international scientific community to assess whether artificial intelligence (AI) algorithms could meet or beat radiologist interpretive accuracy.
“Based on our findings, adding AI to radiologists’ interpretation could potentially prevent 500,000 unnecessary diagnostic workups each year in the United States. Robust clinical validation is necessary, however, before any AI algorithm can be adopted broadly,” said Dr. Christoph Lee, professor of radiology at the University of Washington School of Medicine and physician at the Seattle Cancer Care Alliance. He was the lead radiologist for the Challenge and co-first author of the paper.
[Access downloadable audio/video files, below, of Dr. Lee describing the study's significance.]
Mammography screening is commonly used for early detection of breast cancer. While this detection tool has generally been effective, mammograms must be assessed and interpreted by a radiologist, using human visual perception to identify signs of cancer. This has led to false-positive results in an estimated 10 percent of the 40 million women who receive routine annual breast cancer screenings in the United States.
The findings showed that, while no single algorithm outperformed radiologists, a combination of methods in addition to radiologists' assessments improved screenings' overall accuracy. The research was conducted by IBM Research, Sage Bionetworks, Kaiser Permanente Washington Health Research Institute, and the UW School of Medicine. It involved hundreds of thousands of de-identified mammograms and clinical data from Kaiser Permanente Washington and the Karolinska Institute in Sweden.
"This DREAM Challenge allowed for a rigorous, apples-to-apples assessment of dozens of state-of-the-art deep learning algorithms in two independent datasets,” said Justin Guinney, vice president of computational oncology at Seattle-based Sage Bionetworks and chair of DREAM Challenges.
To help protect data privacy and prevent participants from downloading sensitive mammography data, study organizers applied the model-to-data approach; this avoids distributing data to participants and mitigates the risk of sensitive patient data being released. Participants were invited to submit their algorithms to the study organizers, who developed a system that automatically ran the models on the data.
"The concerns that patients feel about the use of medical images are always first in our minds. The novel model-to-data approach for data sharing is essential to preserving privacy,” said Diana Buist of Kaiser Permanente Washington and co-first author of the paper. “Also, the inclusion of data from two different countries with differing mammography screening practices highlights important translational differences in how AI could be used in different populations."
Gustavo Stolovitzky, director of the IBM Translational Systems Biology and Nanobiotechnology Program and founder of the DREAM Challenges, added, “Our study suggests that an algorithmic combination of AI and radiologist interpretations could provide a mechanism for significantly reducing unnecessary diagnostic workups in the U.S. alone."
The researchers' funding included the National Cancer Institute (HHSN26120110003, P01CA154292, R01CA240403, 5U24CA209923) and American Cancer Society (126947-MRSG-14-160-01-CPHPS).
Downloadable files of Dr. Christoph Lee describing the study's significance:
- Broadcast-quality soundbites (2:31 .mp4)
- Web-embeddable video (2:20 .mp4)
- Soundbite log (.doc)
- Audio soundbites (2:15 .mp3)
- YouTube embed
For details about UW Medicine, please visit http://uwmedicine.org/about.