
Machine learning promises to speed metabolism research
The method reduces differences, often seen between data from different sample sets, that have long hindered progress in the field.Media Contact: Leila Gray - 206-475-9809, leilag@uw.edu
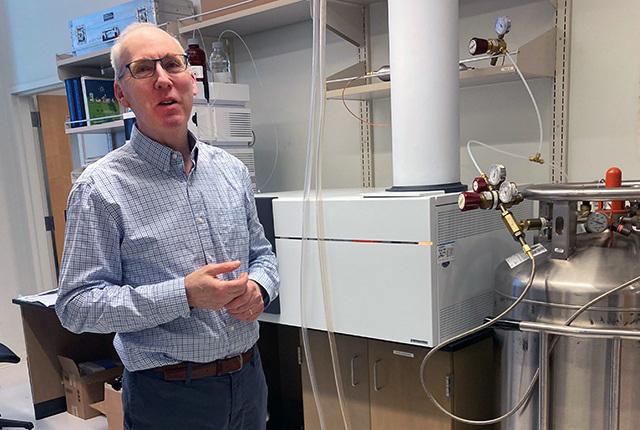
A new study shows that it is possible to use machine learning and statistics to address a problem that has long hindered the field of metabolomics: large variations in the data collected at different sites.
“We don’t always know the source of the variation,” said Daniel Raftery, a professor of anesthesiology and pain medicine at the University of Washington School of Medicine in Seattle. “It could be because the subjects are different with different genetics, diets and environmental exposures. Or it could be the way samples were collected and processed.”
Raftery and his research colleagues wanted to see if machine learning — a form of artificial intelligence that uses computer algorithms to process large volumes of historical data and to identify data patterns — could reduce this variation between data from different sites without obscuring important differences.
“We wanted to bring these mismatched datasets together so the findings of different studies could be compared or combined for further analysis,” Raftery said.
He led the project with Dabao Zhang and Min Zhang, formerly at Purdue University and now professors of epidemiology & biostatistics at University of California, Irvine Public Health. Danni Liu, a Ph.D. student at Purdue, was lead author of the paper, which appears in the Feb.12 issue of PNAS.
Raftery is an investigator at the UW Mitchochondria & Metabolism Center, based at UW Medicine South Lake Union in Seattle.
The term metabolomics relates to metabolism, a word that describes chemical reactions our cells perform to maintain life. These include reactions that break down food to harvest energy and obtain the raw materials cells need for growth and repair, reactions that involve the assembly of cellular components needed for life, and reactions involved in the disassembly of damaged or unneeded components so they can be recycled, discarded or used as fuel.
The small chemicals produced by these metabolic processes are called metabolites. Metabolite levels reveal what chemical reactions are going on within a cell, tissue, organ or organism at a given moment and how those reactions may change over time.
Metabolomics is the study of metabolites and the processes that produce them.
This information helps medical scientists better understand not only how cells maintain normal function but also what might be going wrong when people fall ill. This knowledge could lead to new ways to diagnose, prevent and treat disease, Raftery said.
In the new study, the researchers built machine-learning models to identify factors that were driving the differences between datasets. The models accounted for demographic differences in the study populations, such as age and sex, and used the information contained in other metabolites to explain the observed differences.
The researchers found that their approach reduced the variation between datasets by more than 95% without obscuring meaningful differences, such as those that naturally occur between men and women.
"We’ve shown that our approach has the potential to reduce unwanted variance seen in metabolomic data while retaining metabolomic signals of interest,” Raftery said.
The group plans to expand its studies with the aim of providing a deeper understanding of normal metabolism and identifying biomarkers of abnormal metabolism that can be a sign of disease.
This work was support by U.S. National Institutes of Health grants R01GM131491, R01GM131491-02S2 and P30DK035816.
Written by Michael B. McCarthy
For details about UW Medicine, please visit http://uwmedicine.org/about.