
AI inconsistently assesses cardiac risk from chest pain
Compared with standard assessment tools, ChatGPT-4 was unreliable in interpreting risk from simulated patient data.Media Contact: Brian Donohue - 206-543-7856, bdonohue@uw.edu
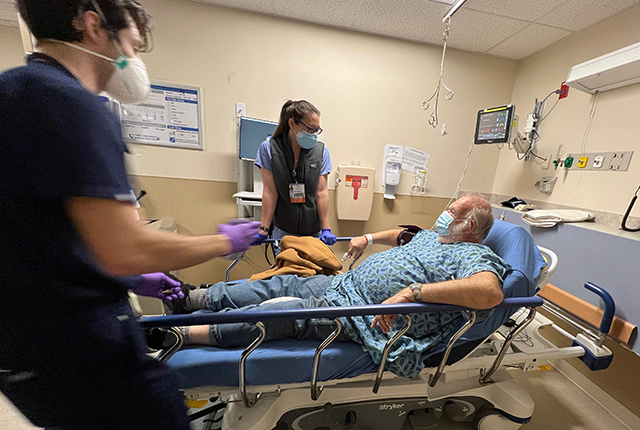
Tasked to interpret data associated with patient complaints of nontraumatic chest pain, the ChatGPT-4 large language model performed poorly against two standard tools that doctors use to predict risk of a cardiac event.
Although the artificial intelligence software aligned generally with the two scoring models (TIMI and HEART), “its inconsistency when presented with identical patient data on separate occasions raises concerns about its reliability,” the authors wrote in describing study findings published April 16 in PLOS One.
The paper’s corresponding author is Dr. Thomas Heston, a clinical instructor in family medicine at the University of Washington School of Medicine in Seattle. Coauthor Dr. Lawrence Lewis is an emergency medicine specialist at Washington University in St. Louis.
“Nearly half the time, ChatGPT gave a risk assessment different from the established clinical tools for the same patient. Highly variable responses in high-stake clinical situations are dangerous and a major red flag,” Heston said.
He described the findings as “a reality check on the hype surrounding medical AI.”
ChatGPT-4 is an internet-based chatbot trained on vast volumes of text data. It is designed to provide speedy, coherent, contextually appropriate responses to queries.
Heston wanted to study its potential to assess nontraumatic chest pain, a common complaint among emergency department patients, many of whom are hospitalized overnight out of caution. The symptom often turns out to have a benign origin, and hospitals have recognized that these complaints are associated with overuse of resources.
“In a high-volume emergency department, you’re just seeing a patient for the first time, but they’re clearly sick and you need to know everything you can about them in rapid fashion,” Heston described. “That’s what ChatGPT is good at. If you could feed in a patient’s full medical record in a HIPAA-compliant way, it ideally would pull out relevant data and red flags in a matter of seconds.”
In this study, ChatGPT-4 was fed bits of information that typically are gathered by emergency department clinicians — for example, aspects of a personal health history, exam, lab test results and imaging studies — and plugged into a model to generate a score that predicts risk. Previous research had independently shown that TIMI and HEART models reliably predict major adverse cardiac events in chest-pain cases.
The researchers created three sets of simulated patient data: one based on TIMI model variables, one based on HEART model variables, and a third that included 44 random variables such as age and pain severity level, but did not include lab test results.
ChatGPT-4 scored each data set five separate times, with its scores compared against TIMI- and HEART-fixed scores.
ChatGPT-4 yielded a different risk score than the TIMI or HEART score 45% to 48% of the time. With the 44-variable model, a majority of the ChatGPT-4’s five scores agreed only 56% of the time, and risk scores were poorly correlated, the authors found.
“Our hypothesis was that ChatGPT would deal with data more like a computer that, when presented with the exact same data, would give the exact same result every time,” Heston said. “What we found was that, even getting the exact same information as TIMI or HEART, ChatGPT would frequently come up with a different risk. If a TIMI score was a lower-risk of 2, ChatGPT would give a risk score of 0, 1, 2, or 3" in its five tries.
“It’s not a calculator. It has this randomness factor,” he continued. “It will treat the data one way and, the next time, treat it differently.”
Heston was encouraged, however, that the chatbot’s responses did not reflect any significant bias based on the simulated patients’ race or sex — that is, the tendency to assign certain populations relatively lower cardiac-risk scores — that other studies have consistently brought to light since the 1990’s.
“That’s the good news from this,” he said. “Our other findings are more of a cautionary tale to all the research out there saying ‘ChatGPT is great.’ Large language models are very good at giving a second opinion but they’re not good at giving a consistently accurate diagnosis.”
For details about UW Medicine, please visit http://uwmedicine.org/about.